Read This If You’re Thinking of Switching into Data Science from Your Existing Career
You‘ve got something valuable we don’t.
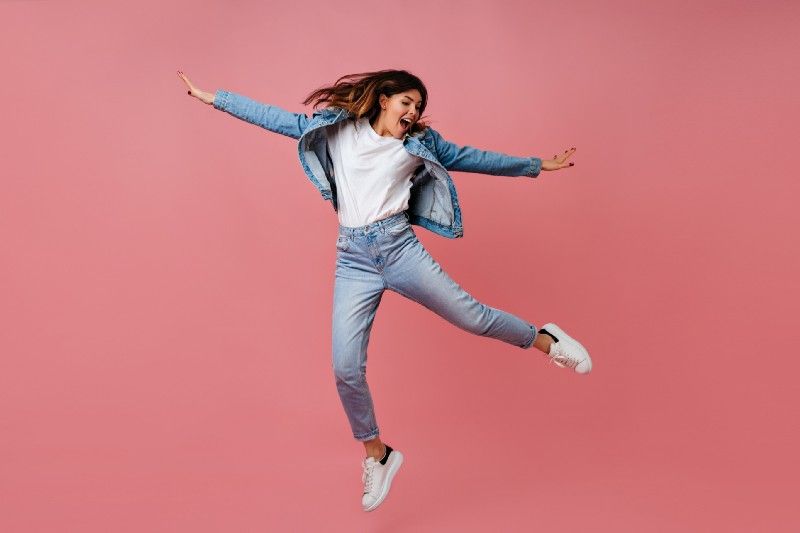
Someone recently reached out to me for some advice, as she has decided to switch her career to data science.
She’s been working in the supply chain space in retail but has been passionate about data science and data-driven decisions. She’s been thinking of making the switch but had multiple concerns regarding the transition.
- She felt she’d have to start again from scratch as she switched into a new role in her career.
- Since the field is ever-evolving, she’ll have to learn and acquire a significant amount of skills to make the transition.
- She felt she might have to settle for lesser compensation because even the fresh graduates are skilled and are ready to work for lesser compensation.
Now, I’m sure you also have similar concerns. These are definitely valid, and a wrong decision would ruin your career into peanuts. Imagine if you do all the hard work and realize it’s not what you expected and not worth it.
As we spoke further, she revealed something that shocked me to the core. She’s been working in the retail space for 12+ years, and all I have is 3+ years. My imposter syndrome kicked in, and I wasn’t sure if I should even offer advice.
If you’re in a similar situation (even if not as long as 12 years), I’m here to tell you there’s light at the end of the tunnel. So stay with me as I take you through I what I told her.
We See Something in You — We Don’t Know What It Is
But if you allow me, we can find that together.
I don’t have 12+ years of experience in any domain, let alone retail. None of my colleagues have.
To put this into perspective: 99.9% of data scientists don’t have that kind of experience. Even the data science hype came into play less than 10 years ago.
According to the data science Venn diagram, unlike other fields, data science combines computer science, maths/statistics, and domain expertise. So, depending on the career you’re coming from, you’d possess at least one of the skills.
In other words, my question to you: What is the strength you already possess from your existing career? Pause and think about it.
Data science has applications in most domains; if you’re coming from health care, social sciences, economics, software engineering, marketing, accounting, and whatnot, AI and data science progress in all of these.
You must be aware of these advancements specific to your area of expertise. Interviewers and business leaders are highly likely to hire you only if you can convince them that your domain expertise can easily be converted into a data science skillset.
As our conversation proceeded, I helped her figure out that she has the utmost level of domain expertise in the supply chain, which most data scientists (including me) lack.
Her next steps would be to research data science applications in the supply chain and familiarize herself with those concepts.
She’d even use her expertise to ideate possible solutions to common problems such as demand planning, supply chain optimization, and more.
On to the next concern.
Upskilling Is Inevitable — But Isn’t It What You Wanted
Look, if you don’t do this right — you’ll regret the switch altogether eventually.
If you had heard that AI and data science are a rapidly evolving field and you need to learn new stuff continually, you heard it right.
You know what? We love doing that. Most data scientists are passionate about what they do, and that drives them to deliver beyond expectation.
If you’re thinking of a switch, you should be prepared to go out of your comfort zone and constantly upskill yourself. Beyond your existing domain expertise, you still need to acquire computer science, machine learning, and statistical skills.
If you don’t enjoy the process of learning them, that’s a red flag.
You might want to reconsider if you really want to make the switch. We don’t claim it’s for everyone, and there are definitely other equally meaningful and enjoyable jobs.
If you feel like I’m all of a sudden discouraging you, yes, I am. However, I want you to resist my discouragement and continue further because you really want to do this for the right reasons.
So that’s exactly what I told her. Isn’t this what you wanted to do anyway? So please take the time to go through some courses, read a few books, and see if you enjoy it. There’s no hurry and be sure before you take a call.
On to the biggest concern of all.
You Don’t Have to Settle for Lower Compensation
Some people may claim otherwise. We call them “wrong.”
The rationale for this concern was: “Fresh data science graduates enter the market every year. Why should the employer hire a complete rookie like me for a higher salary if they can get a fresh graduate for a lesser salary?”
I had a chuckle when I heard this. Everything’s fair, except for comparing you with a fresher.
A fresher has no industry experience and is typically guided by the seniors. But you, my friend, have years of industry experience already.
You know how projects are managed. You know how to handle meetings and clients. Some of you know how to market the products and even more. So you know a lot of stuff, even we (as data scientists) do not know.
The employer should be aware of this. If they aren’t, you must showcase your capacity and make them realize it.
Once they understand that you would adapt to become a full-fledged data scientist within a year, you’d be much more valuable than a fresher.
As we spoke further, we decided her next steps would be to list down the AI and data science companies that focus on supply chain space and approach them. This simple strategy would maximize her chances of a quick switch.
If you've identified your transferable skills, research the intersection of your existing career and data science, slowly upskill yourself, you’d naturally be able to convince your potential employer of your worth.
How to Make the Transition Work for You
Here’s the part where we give actionable takeaways.
- If you’re coming from another career, chances are you already have some transferable skills. Identify your strengths.
- Focus on your strengths, and slowly acquire the rest of the skills.
- Research the intersection of your domain and AI/Data science to understand the common problems that need to be solved.
- Ideate or familiarize with few probable solutions to those problems.
- Go on a continuous learning mode. If you don’t enjoy this, you probably won’t enjoy the data science career as well.
- Showcase your domain expertise and adaptability as your strength to negotiate competitive compensation.
Will the transition be easy? No. Would you enjoy it once you’ve done it? Hell yeah. Look, you’re not alone this; many are doing this.
You might even have more concerns and queries, let me know in the comments, and I’d try to answer them as well. If I can’t, I’d at least ask my colleagues who had done it themselves.
Everyone is willing to help you cross the bridge. Will you do it?
I say you can. The data science community says you can. Prove us right.
For more helpful insights on breaking into data science, honest experiences, and learnings, consider joining my private list of email friends.